AI in Mental Health.
Imagine a world where your phone knows you’re depressed before you do. Sounds surreal, right? But it's already happening. AI is increasingly being used in mental health care, leveraging everything from your social media posts to your typing patterns.
With Artificial Intelligence (AI) making waves across so many fields it's no surprise that researchers have taken to how AI can improve/provide mental health support. This is especially prudent given the lack of clinicians for the number of patients (in the UK). Technologies like Natural Language Processing (NLP) and machine learning have been tested in the hopes of revolutionising how mental health disorders are detected, diagnosed and treated. However, whilst there are these groundbreaking advancements that take the highlight an element of caution is also needed or at least to the extent of using that caution to reduce risks such as privacy concerns and the ethics involved.
Ok, so what can AI do? Well, contemporary AI involves the use of algorithms and data analysis which are used to analyse a huge amount of data to be able to predict and therefore detect mental health disorders, sometimes before symptoms even appear. This is done by examining digital footprints and behavioural patterns to infer an individual's mental health status where that individual can then be forwarded into early intervention. AI can also provide personalised care without having to see someone in person which is super beneficial when you have a too small workforce of clinicians.
Additionally, related to the pandemic, according to a survey by the American Psychological Association (APA), 84% of psychologists who treat anxiety disorders say there’s been an increase in demand for treatment since the start of the pandemic, which is up from 74% a year earlier.
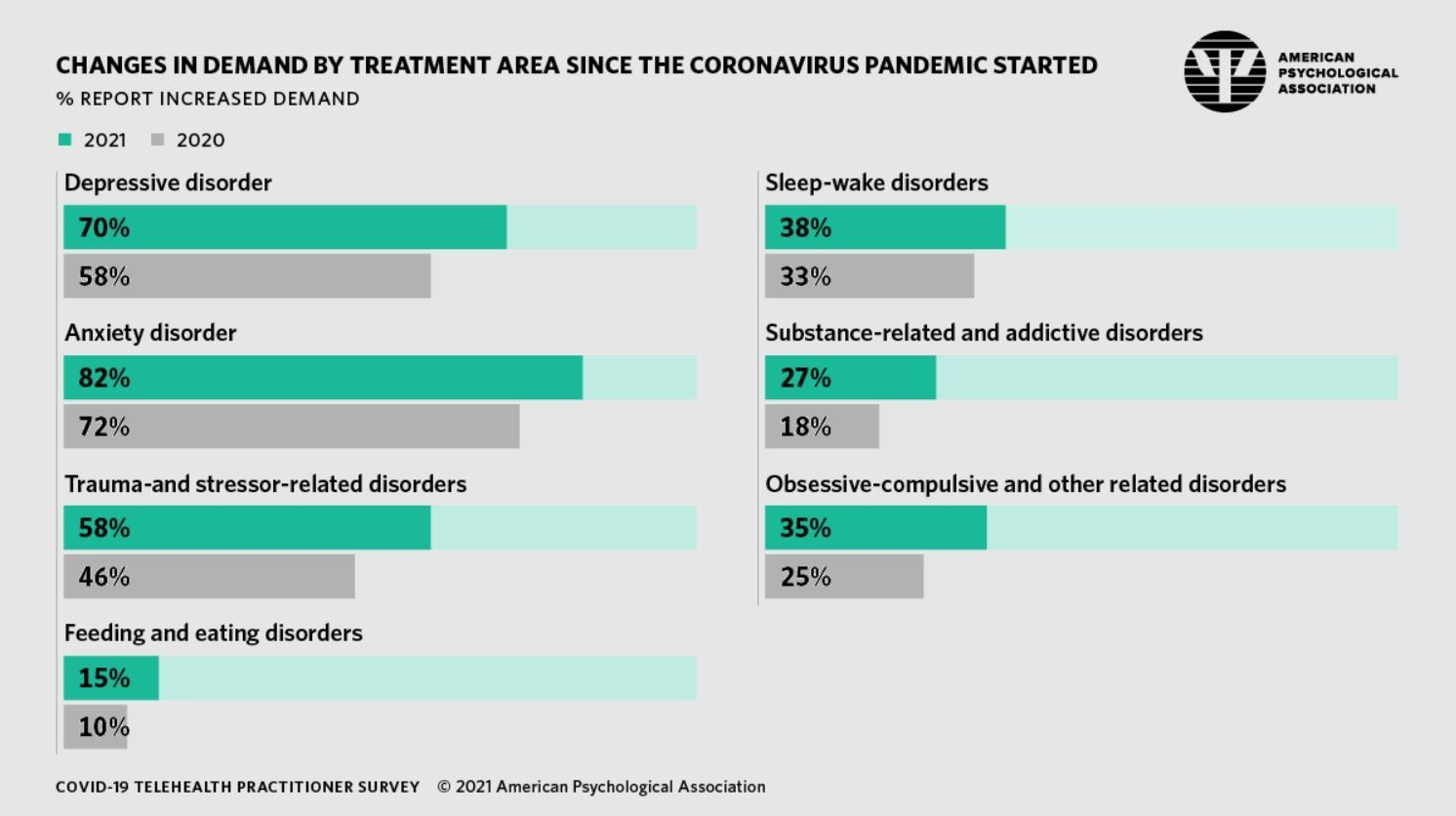
Part One: Applications
Predictive Analytics and Early Intervention
AI algorithms are increasingly being used to predict mental health crises. For example, machine learning models can analyze vast datasets from electronic health records, social media, or wearable devices to identify individuals at high risk for conditions like depression or anxiety. The ability to detect these signs early allows for timely interventions, potentially preventing crises before they escalate (WHO).
Virtual Therapists and Chatbots
AI-driven virtual therapists are gaining popularity as tools that offer immediate mental health support. Unlike traditional therapy, which can have long wait times and high costs, AI chatbots provide an accessible alternative for people seeking help. These tools use natural language processing to deliver cognitive-behavioural therapy (CBT) techniques and are particularly effective in reaching underserved populations, such as non-binary and ethnic minority groups, where accessibility and cultural competency are critical (Augnito.ai). They are also super useful for people in more rural areas who might not have easy physical access to a therapist nor good enough internet access for video calling. This is where chatbots are super useful along with providing a feeling of greater anonymity for users which can make people want to share more if they aren't talking face-to-face (although there are many advantages to face-to-face)
Precision Medicine and Personalized Care
Mental health care is inherently complex and highly individualized. Recent research emphasizes that AI can support a precision medicine approach, which tailors treatment based on the unique triggers and needs of each patient. For example, smartphone-based AI tools can monitor behavioral data, such as phone usage patterns, to provide insights into mental health conditions. However, the effectiveness of these tools varies significantly based on individual contexts, indicating the need for more personalized AI applications in mental health (Medical Xpress).
AI in Diagnostic Processes
AI has demonstrated potential in enhancing diagnostic accuracy by analyzing data more effectively than traditional methods. AI algorithms can process data from diverse sources—such as clinical observations, patient self-reports, and even genetic information—to provide a more comprehensive understanding of a patient's mental health. However, the integration of AI in diagnostics comes with ethical concerns, such as maintaining transparency and avoiding biases in AI-driven decisions (Frontiers).
Part Two: Risks
While the potential of AI in mental health care is vast, it is not without challenges. The complexity of mental health conditions makes it difficult for AI systems to fully capture the nuances of individual experiences. A study from Cornell Tech highlights the difficulties in creating AI models that accurately predict mental health conditions due to the deeply personal and subjective nature of mental health. For example, high smartphone use might indicate depression in older adults but could suggest a lack of engagement in younger populations (Medical Xpress).
Another significant challenge is public trust. A study by the British Standards Institution (BSI) found that while half of respondents support using AI in healthcare to reduce wait times, a significant portion still does not trust AI systems. Concerns include data privacy, the potential for biased algorithms, and the dehumanization of care. For AI to be effective in mental health care, it must be developed with transparency, inclusivity, and a deep understanding of the ethical implications (Frontiers).